Various applications of neural networks and complex engineering and physical simulation, such as climate modelling, use global optimization techniques which consist in finding the global optimal solution of a given objective function with potential multiple local optima. The ability to guarantee convergence to the global optima is in general a very hard task.
A particular family of problems belongs to the bound-constrained global optimization of functions with low effective dimensionality, which only vary over a subspace and are constant along a linear subspace. However, the reduction of the problem to its effective subspace is not immediate as the constant subspace is generally unknown.
The authors propose a generic algorithm framework, which repeatedly solves reduced subproblems within random low-dimensional subspaces using any global, and even local, solver in the subproblem. In particular, one such solver being employed and tested is Artelys Knitro which the authors have found to be flexible and easy to use.
Start with a tutorial!
You’re not familiar with nonlinear optimization? This tutorial will present some examples of nonlinear problems for various applications. You will discover nonlinear programming methods using the Artelys Knitro solver in a Python notebook, through different examples.
Free trial
Get your trial license to test Artelys Knitro’s performances on your own mathematical optimization problem. The trial package includes free support and maintenance. You can have access to Artelys Knitro for free with a 1-month unlimited version or a 6-month limited version.
Artelys Knitro has unmatched performance
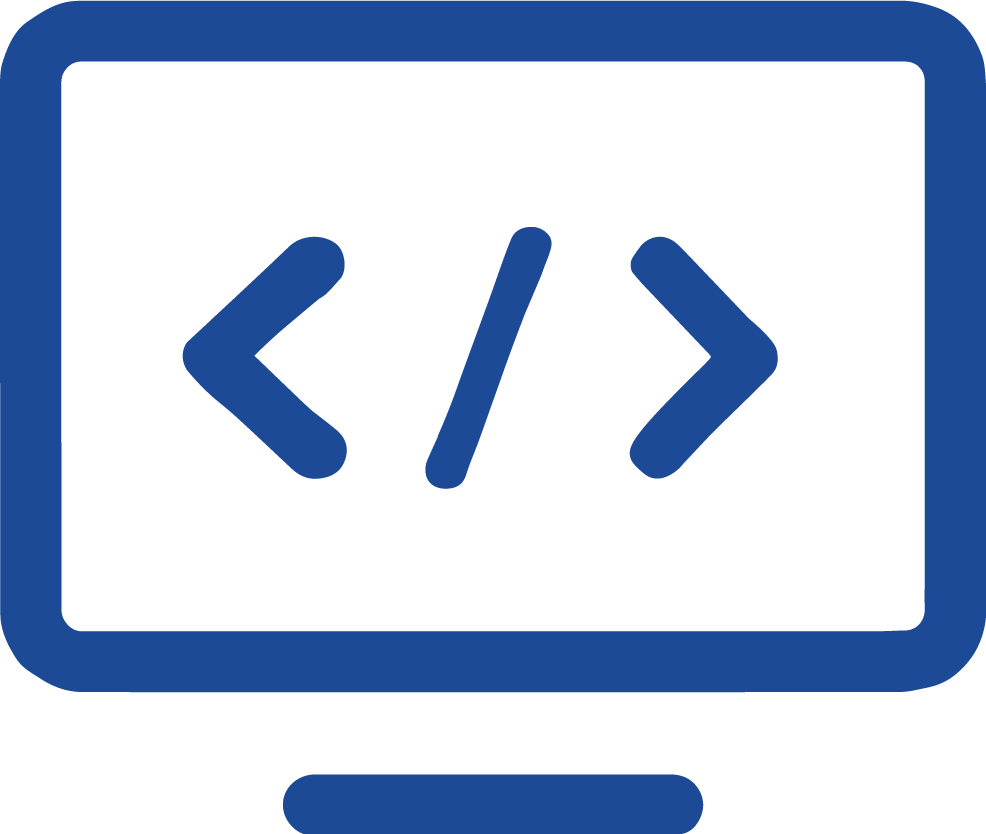
Best Nonlinear Solver
Artelys Knitro has been ranked every year by public benchmarks consistently showing Artelys Knitro finds both feasible and proven optimal solutions faster than competing solvers.
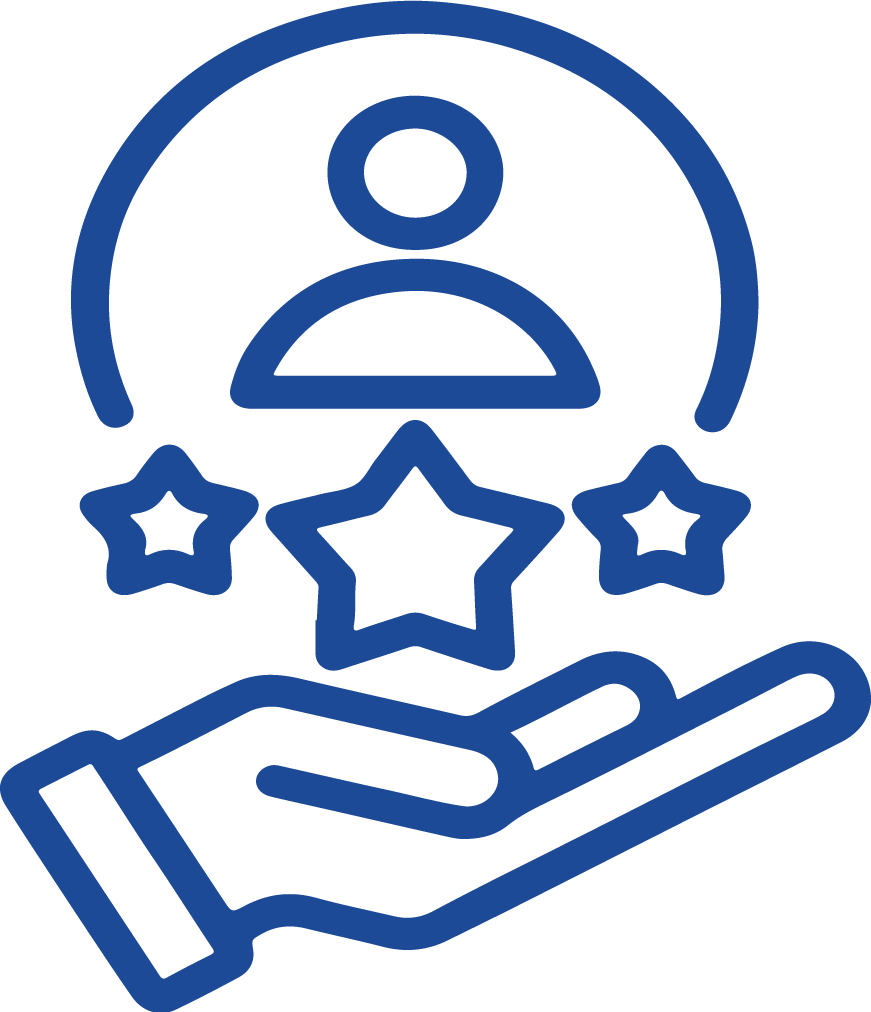
Technical support
The Artelys technical support team comprises Artelys’consultants (PhD-level) who are used to solving the most difficult problems and deploying enterprise-wide optimization solutions. They can advise on algorithmic or software features that may result in enhanced performance in your usage of Artelys Knitro.
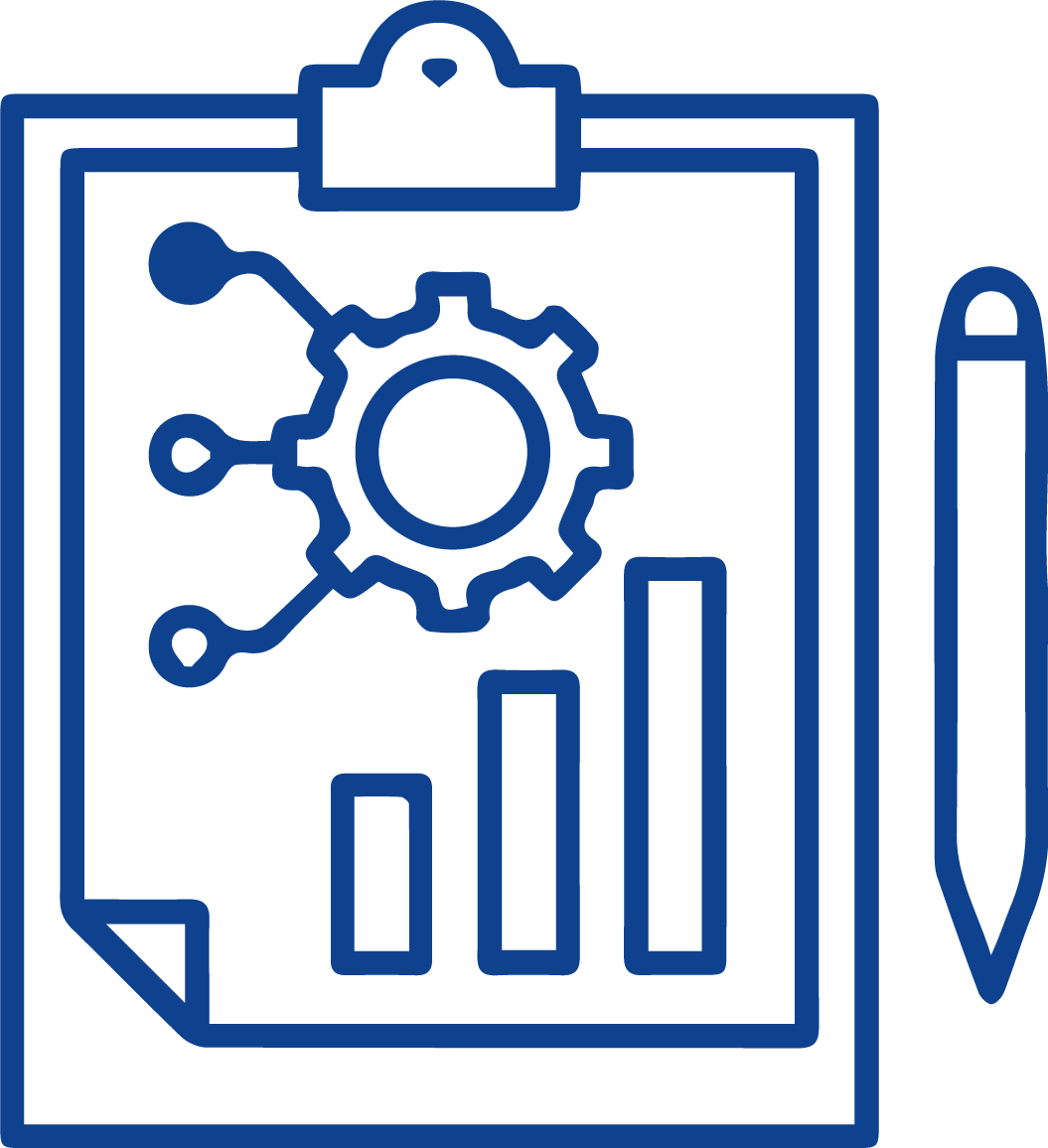
Updates and new features
The development team works continuously to provide two releases of Artelys Knitro every year. Based on feedback, we always improve our solver to meet users’ requirements and need to solve larger models faster.
© ARTELYS • All rights reserved • Legal mentions