Artelys Knitro is now available on ARM and opens up to the embedded world
With the development of smartphones, IoT and complex automated systems such as autonomous vehicles, there are more and more opportunities to integrate sensor-driven machine learning and optimization into embedded systems.
These technologies are widely used in AI, but why exactly use a nonlinear solver into embedded systems?
The solver may be used during the training phase of a Machine Learning model
For instance, a vision system that detects defects on a production line may have learnt from a set of data comprised of both compliant and faulty products. In practice this training involves the adjustment of many parameters in order to minimize the error rate. This often means solving a nonlinear optimization problem.
During the training phase of the model, many parameters are chosen in order to minimize a nonlinear objective function comprised of both the error and a regularization term.
In many cases, the training phase is carried out in the cloud, and the trained model is used to perform inference on the device. However, this process is slow because of the time needed to transmit the data and model updates between the device and the server. In situations where the device is expected to learn and respond to the new scenario instantly, there will be a need for local model updates. Local training also addresses the important issue of privacy, as the data does not need to leave the device.
Some applications require to perform real-time numerical optimization
Self-driving cars integrate machine learning and optimization in a complimentary way. The first enable the system to learn how to identify static and moving objects, as well as signalization.
Then the vehicle must compute a trajectory towards the desired location that maximizes safety and comfort while minimizing energy consumption and travel time, taking into account obstacles, signalization, road shape… This is a nonlinear optimization problem. And there are many more examples of embedded optimization such as drone stabilization or rocket landing!
Knitro 12.2 is available on demand for ARM processors (ARMv7 (32 bits) and ARMv8 (64 bits)). Moreover, for the enthusiasts, Knitro is available for Raspberry Pi (ARMv7, Raspbian 9).
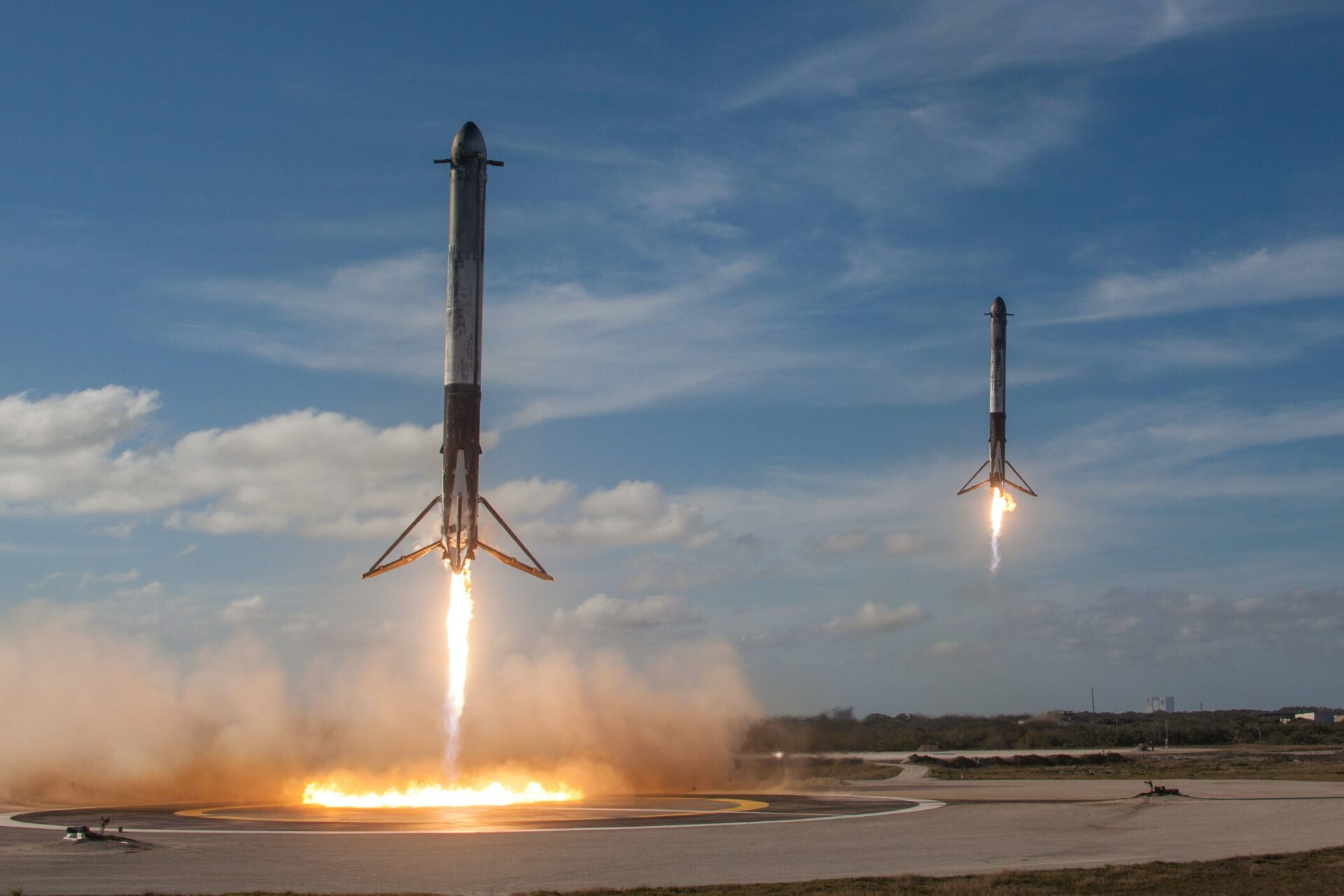
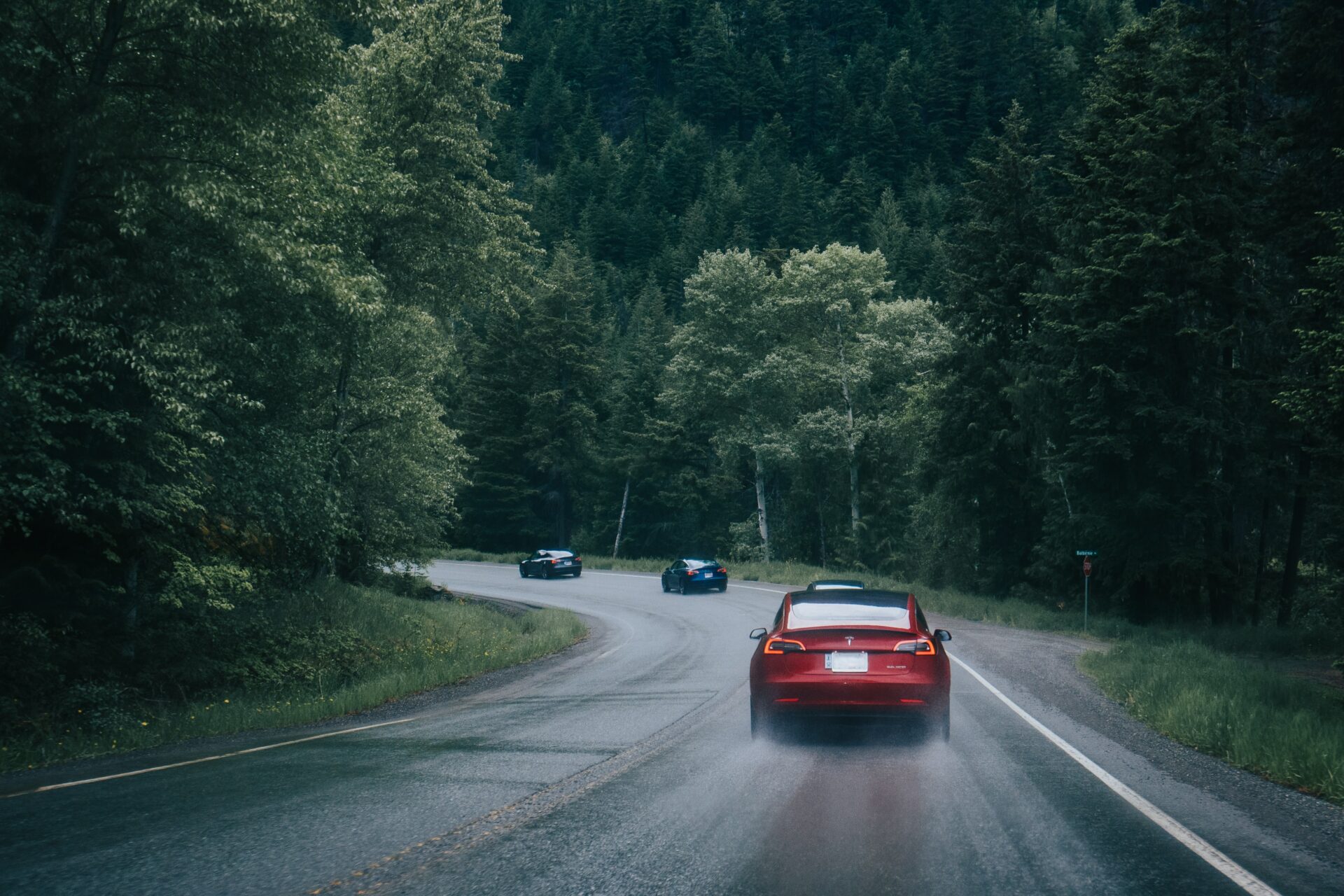
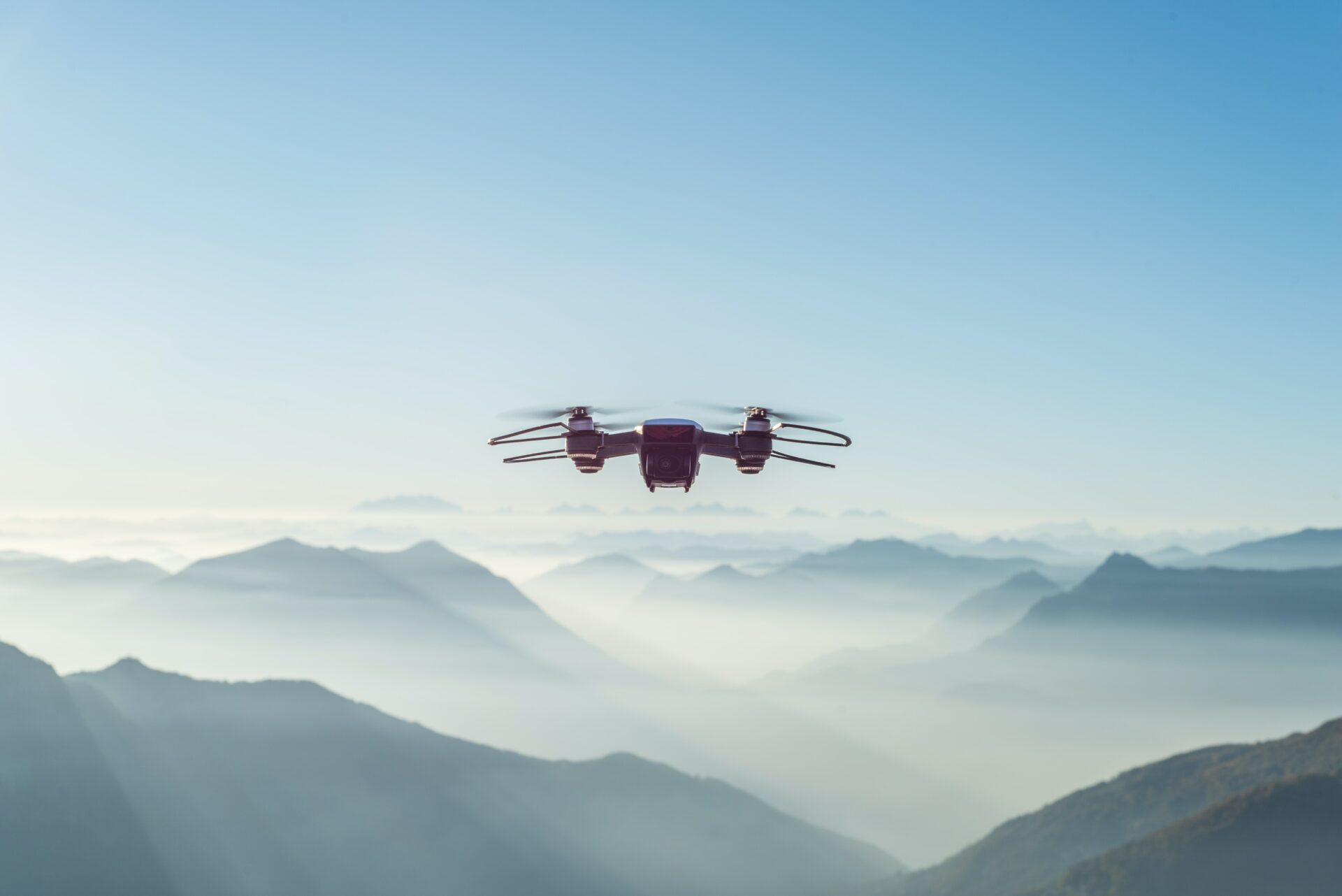
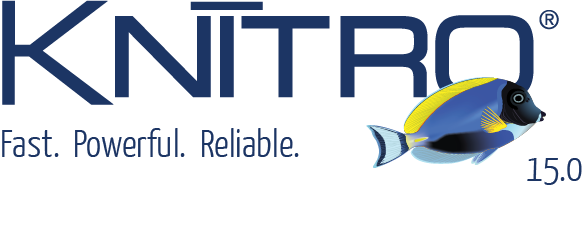
Artelys Knitro 15.0: New Tools for Your Large-Scale Models
Artelys is pleased to announce the release of Knitro 15.0, which provides new algorithms and performance improvements to solve your large-scale optimisation problems, whether linear or non-linear, more quickly.
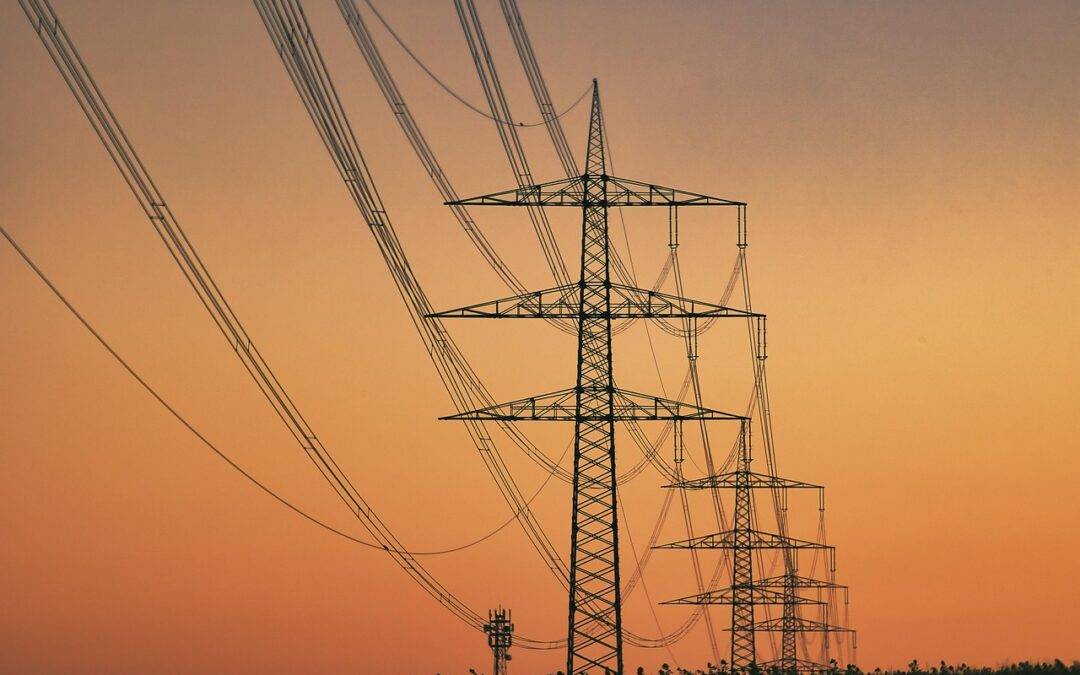
Artelys Introduces Future Sight: a Visualisation Tool Supporting the Energy Transition
As partner in the European Climate + Energy Modeling Forum (ECEMF) – a Horizon 2020 Europe project uniting research institutes and leading energy modellers in Europe – Artelys has contributed to modeling activities powered by Artelys Crystal Super Grid modelisation tool, and has led the development of a fully-fledged visualisation tool.
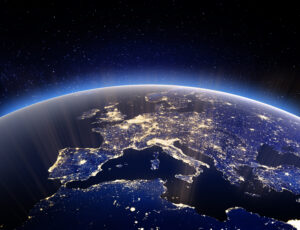
Supercharging Optimisation: How Artelys, FICO and NVIDIA cuOpt Join Efforts to Scale Up Energy System Optimisation
As energy system models continue to scale—reaching up to hundreds of millions of variables and constraints—traditional CPU-based optimisation solvers are hitting performance and memory bottlenecks. These increasingly complex models are essential for planning Europe’s energy transition, yet solving them within realistic timeframes has become a pressing challenge.
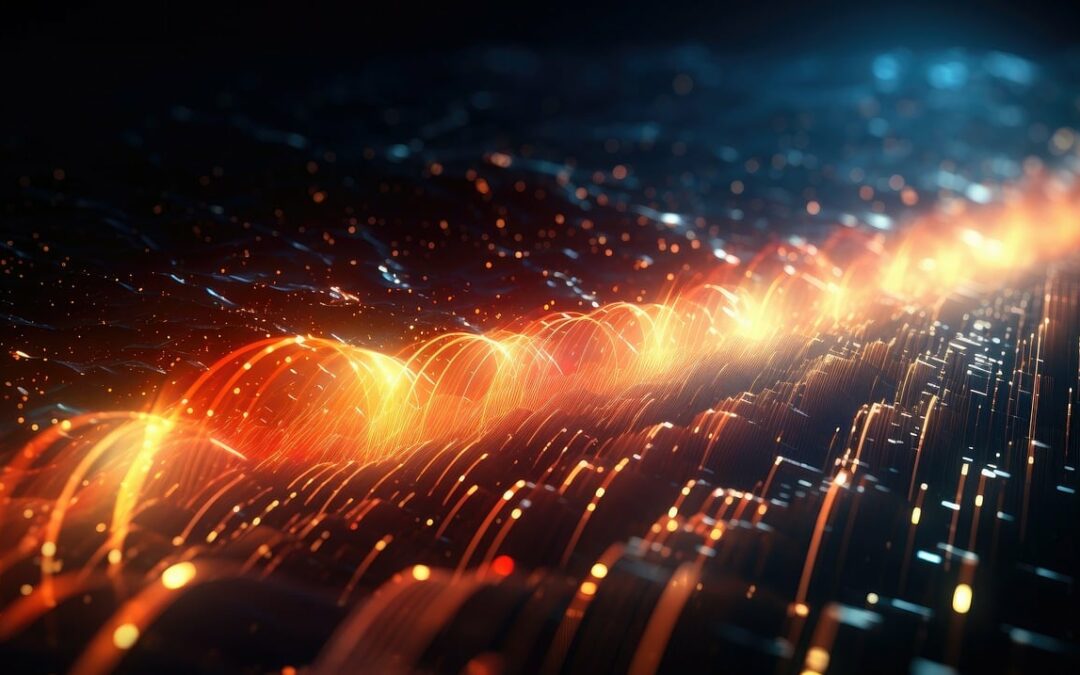
Artelys is powering SEleNe CC’s Common Grid Model (CGM) Service
Artelys provides maintenance and support services of the calculation module used to perform the European Merging Function (EMF) at SEleNe CC
subscribe to our newsletters
© ARTELYS • All rights reserved • Legal mentions